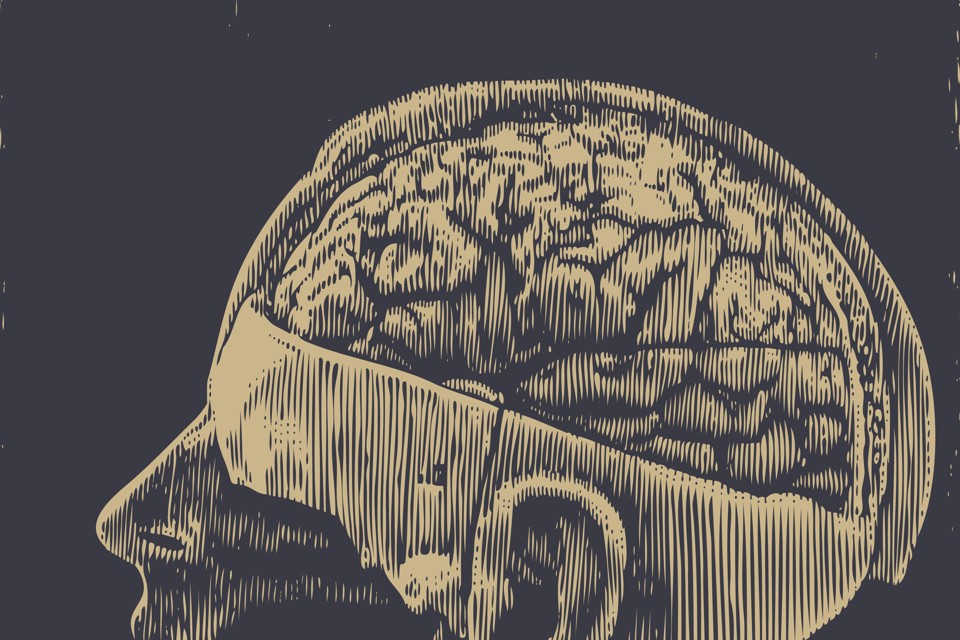
The Human Remembering Machine
A new mathematical model of memory could accelerate the quest to build super-powered, brain-inspired hardware systems.
The Atlantic | 3 October 2016
They called it the Hubble
Telescope of the mind.
This was in 2009, after the announcement that a team of scientists from IBM’s
Cognitive Computing group had built what was, at the time, the largest
artificial brain ever. It was a cell-by-cell computer simulation of the human
visual cortex, large as a cat’s brain.
The reference to Hubble, the
deep-space telescope, is a nod to the galactic complexity of building a
computer with brain-like infrastructure. The cat-sized brain built in 2009
represented 1 billion neurons connected by 10 trillion synapses, according to IBM. Since
then, they’ve scaled up dramatically—mapping the neural pathways of a macaque
monkey brain, and edging closer to an accurate simulation of the human brain.
Simulating an entire,
biologically realistic human brain remains an elusive goal with today’s
hardware. The processing power that would be needed to pull off such a feat is
mind-boggling. “It would be a nuclear power plant,” Horst Simon, a
mathematician and the deputy director of the Lawrence Berkeley National
Laboratory, told Popular
Mechanics in
2009. “The electricity alone would cost $1 billion per year.” Since then,
scientists have said they expect to be able to simulate a human-scale brain by
2019, but they still haven’t solved the problem of how to power such a
simulation. (That said, Simon and others have successfully created computer
simulations inspired by the number of synapses in the human brain—which is
different than a biologically realistic model, but still one step toward that
ultimate goal.)
Artificial brains are such energy hogs because
they can be infinitely precise, meaning they can draw on colossal troves of
data to do what they do. Consider, for example, a neural network used for
pattern recognition—the kind of system that’s trained on a massive database of
images to be able to recognize faces. The humongous dataset required to train the
system is what makes it effective, but it’s also what prevents efficiency. In
other words, engineers have figured out how to build computer systems that have
astonishing memory capacity, but they still need huge amounts of power to
operate them.
This is a problem for anyone
who wants the technology behind a brain-inspired computer to be widely
available, scalable down to the kinds of devices—say, smartphones—that ordinary
people actually use. This scaling problem also helps explain why scientists are
so interested in building computers that mimic the human brain to begin with;
human brains are both highly sophisticated processors—people carry around a
lifetime of memories, after all—and they are remarkably energy-efficient.
If engineers can figure out
what makes a human brain run so well, and on so little energy relative to its
processing power, they might be able to build a computer that does the same.
“But that has always been a
mystery,” says Stefano Fusi, a theoretical neuroscientist at Columbia
University’s Zuckerman Institute. “What we wanted to understand is whether we
can take advantage of the complexity of biology to essentially build an
efficient [artificial] memory system.”
So Fusi and his colleague, Marcus Benna, an
associate research scientist at the institute, created a mathematical model
that illustrates how the human brain processes and stores new and old memories,
given the biological constraints of the human brain. Their findings, published
today in a paper in the journal Nature
Neuroscience,
demonstrate how synapses in the human brain simultaneously form new memories
while protecting old ones—and how older memories can help slow the decay of
newer ones.
Their model shows that over
time, as a person stores enough long-term memories and accumulates enough
knowledge, human memory storage becomes more stable. At the same time, the
plasticity of the brain diminishes. This change helps explain why babies and
children are able to learn so much so quickly: Their brains are highly plastic
but not yet very stable.
“That’s why there is a critical
period for many abilities like learning languages,” Fusi says. “As you
accumulate knowledge, it becomes extremely difficult to learn something new,
much more difficult than it is for kids. That’s certainly reflected by any kind
of model like ours, where you essentially have what is called metaplasticity.”
The new model, inspired by how the brain
actually works, imitates the plasticity of human synapses over time—and the way
older memories affect the storage of newer ones. “In our case, by combining
together all these different variables in the model,” Fusi says, “we can extend
the memory lifetime without sacrificing the initial strength of the memory.
That is what’s important.”
The significance of the latest findings go
beyond a theoretical interest in simulating biological systems more accurately,
which in and of itself could provide a crucial new framework in neuroscience.
In addition, such simulations hint at the real possibility of a new class of
neuromorphic hardware powered by supremely powerful and surprisingly small
computers.
The model allows for a “much more efficient way
in terms of energy,” Fusi says, “so if you want to integrate this [artificial]
brain technology—into your mobile phone, so your mobile phone can drive your
car for you, you’re probably going to need this kind of computer.”
No comments:
Post a Comment